The Evolution of Generative AI Through the Lens of NLP: From Word Embeddings to ChatGPT
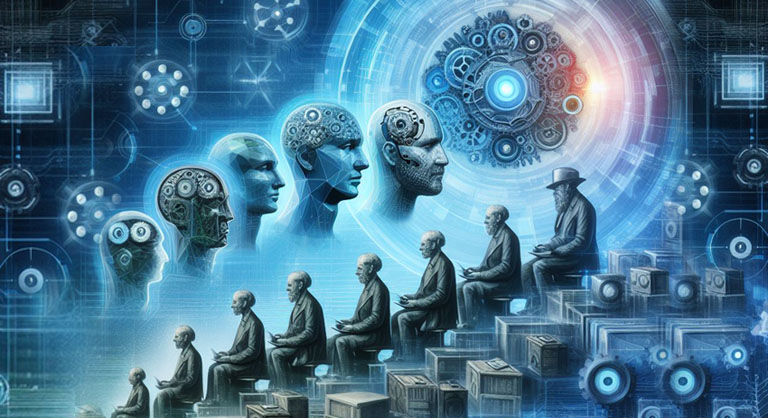
The landscape of Natural Language Processing (NLP) has evolved dramatically over the years, marked by innovative models and breakthrough techniques. This journey from foundational methods like word embeddings to the latest generative pre-trained transformers (GPT) reflects the leaps in machine understanding and language generation. Here’s a quick overview of how NLP technologies have progressed, ushering us into a new era of human-machine communication.
Foundational Steps: Word Embeddings and Attention Mechanisms
The inception of advanced NLP can be traced back to 2013 with the advent of word2vec, a model introduced by Google that transformed words into dense vectors based on contextual relationships. This was revolutionary as it captured semantic nuances that older models couldn’t grasp. However, as significant as word embeddings were, they couldn’t focus on different parts of text to form a larger understanding. This limitation was tackled by attention mechanisms, which were introduced in the 2017 paper “Attention Is All You Need.” These mechanisms led to the Transformer architecture, the backbone of many later models, which allowed models to form understanding of text beyond words and sentences. Together, word embeddings and attention mechanisms laid the groundwork for a new generation of NLP technologies.
Transfer Learning and Contextual Understanding: ULMFiT Meets BERT
Transfer learning in NLP truly took off with ULMFiT in 2018. Developed by fast.ai, ULMFiT made it possible to fine-tune a general-purpose language model to specific tasks. This was a game-changer as it significantly reduced the data and computational power required for state-of-the-art performance. In the same year, Google released BERT (Bidirectional Encoder Representations from Transformers), which upped the ante by integrating the Transformers architecture over ULMFiT’s LSTM (long short-term memory) architecture. BERT and ULMFiT collectively shifted the focus from understanding individual words to grasping the intricacies of sentences and paragraphs.
The GPT Era: From GPT to GPT-3
Initially introduced in 2018 by OpenAI, the Generative Pre-trained Transformer (GPT) models marked a shift in the landscape. While GPT-1 was impressive, GPT-2 released in 2019 caused quite a stir due to its ability to generate human-like text, so much so that OpenAI initially withheld its full version from public release. By 2020, GPT-3 was unveiled, boasting 175 billion parameters and setting new benchmarks in a range of NLP tasks. These models signified not only advancements in generating text but also a rethinking of how machines could understand and engage in human conversation.
The Advent of ChatGPT
Building on its lineage of GPT models, OpenAI introduced ChatGPT, a specialized version of GPT-3 designed for conversational agents. Unlike task-specific bots, ChatGPT aims to engage in open-domain dialogue, capable of discussing a wide variety of topics. Its versatility and capabilities make it a frontrunner in the world of automated customer service, online moderation, and even as a creative tool for writers and developers.
The Road Ahead
As we continue to push the boundaries of what is possible in NLP, models are becoming more than just tools; they are active participants in generating information, creative content, and solutions. With each advancement, the potential applications for NLP in both specialized and general contexts continue to grow, shaping how we interact with technology and even with each other.
The journey from word embeddings and attention mechanisms to state-of-the-art models like ChatGPT illustrates the monumental strides that have been made in understanding and generating human language. As we look forward to the next milestone, one thing is clear: we are only scratching the surface of what is possible in the realm of NLP and generative AI.